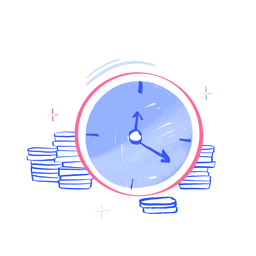
We could not find any results for:
Make sure your spelling is correct or try broadening your search.
Name | Symbol | Market | Type |
---|---|---|---|
1x Tsla | LSE:TSLA | London | Exchange Traded Fund |
Price Change | % Change | Price | Bid Price | Offer Price | High Price | Low Price | Open Price | Traded | Last Trade | |
---|---|---|---|---|---|---|---|---|---|---|
-11.13 | -2.61% | 414.425 | 398.40 | 430.50 | 461.575 | 378.575 | 423.05 | 3,534 | 16:35:12 |
Date | Subject | Author | Discuss |
---|---|---|---|
29/4/2024 12:55 | Up 18 in the premarket! Is this permission in China really worth $55 Bn? | ![]() hosede | |
29/4/2024 11:51 | H, As well as allocating capital and setting strategic goals, he's a brand ambassador. Branson was brilliant at this, creating fun, inspiration and aspiration. Jobs was good at inspiration and aspiration. What does Musk offer? Doxing, ranting and conspiracy theories! | simon gordon | |
29/4/2024 11:40 | We'll have to see how the market reacts to this move in early trading. If it is sold into then it means large holders are continuing to distribute. | ![]() hpcg | |
29/4/2024 11:30 | Musk is deluded in the sense that he thinks he is adding IP to the company, as implied by what he said about AI development. The external world, or at least the entertainment industry, still has a concept of a lone wolf scientist / engineer / developer making the big steps forward. It just doesn't happen. Even breakthroughs made by individuals sit on the framework or published papers and research that they have imbibed, and the conversations and ideas they have had with peers. As CEO he is allocating capital and setting strategic goals. In that context the Cybertruck has been a disaster. Then we have the humanoid robot, which I am reasonably certain results from Musk's resentment at paying any workers. The conflict with his now former senior lieutenants over strategy is another red flag. They will be much closer to the reality of where robotaxis are. I would imagine almost no one reports up bad news, or if they do it stops at the level below Musk. He is very unlikely to be making decisions on the full facts. I guess the only way of investing in BYD is through Berkshire Hathaway which has 8%. The trouble is even if BYD becomes a $2tn market cap company that only adds 15-20% to BRKs market cap. | ![]() hpcg | |
28/4/2024 22:15 | Par for the course Monty | ![]() hosede | |
28/4/2024 21:33 | I think it's all going to end in tears. | ![]() montyhedge | |
28/4/2024 21:21 | Bluster, The compos mentis of the people in leadership positions in a company is paramount. A red flag in AGFX was Digby Jones as Chairman, some would say I am negative on him because of Brexit or his politics. To me, it was his utterances that made me question his leadership position. I think it was Musk's hyping of crypto that made me start to pay attention to his utterances. Was it dogecoin. Some business analysts think he's a huckster and a charlatan. Some a gambler who got lucky. Others think he is a genius. My business heroes are humble, like Ernest Harrison at Racal. The BYD leader looks pretty switched on and is moving ahead of Tesla in making mass-market EVs. | simon gordon | |
28/4/2024 21:08 | Hi Bluster, My interest in Tesla is the business drama. Musk's political diatribes are an angle in the story. He looks to have lost his mind on Twitter and now some owners are putting bumper stickers on their cars and the Tesla brand is being damaged. That's bad business. | simon gordon | |
28/4/2024 21:03 | Good to see you posting again, Simon. I did wonder whether or not your interest here was political - thanks for confirming. “Musk had it all, then lost his mind on Twitter. Wang Chuanfu is getting on with creating a clean energy behemoth.“ | ![]() blusteradjuster | |
28/4/2024 18:15 | hpcg: Having gravity working in your favour makes it a hell of a lot easier! | ![]() cfb2 | |
28/4/2024 18:11 | hosede: The maximum size of the neural network in each car's FSD computer is the same, rather like the RAM in your computer. When Tesla is putting new training data into their neural networks for FSD they are just changing the numbers; the overall data size does not change. This is not like going for a new version of software when you'd expect the software to grow in size with all the extra features they have added. The effect described in the article by Will Lockett according to Sam Altman is real. The relationship between the number of training cycles is not proportional to the quality of data. Eventually you end up with, say, doubling the training and getting only a 1% increase in the reliability of the data. If you're trying to recognise whether a picture contains a cat or a dog with a 100% accuracy this is a huge problem. Does this mean that robotaxis will plateau and never be made to work? No. If you remember, the cars are using video data; there is a time element and movement involved. If the cars are putting through video frames every 20ms they get multiple attempts at interpreting the data at different angles. | ![]() cfb2 | |
28/4/2024 18:07 | There was a superb piece on BYD in the FT last week. “I don’t think people realise BYD’s greater ambition is to be an energy ecosystem company,” says Bridget McCarthy, the head of China operations at Snow Bull Capital, a Shenzhen-based hedge fund invested in BYD. Selling passenger vehicles is just the first step, she adds. “They’re trying to say: ‘we’ll electrify your fleets of commercial vehicles, we’ll give you the energy storage, we’ll give you solar so you can generate electricity’.& ===== Musk had it all, then lost his mind on Twitter. Wang Chuanfu is getting on with creating a clean energy behemoth. | simon gordon | |
28/4/2024 17:48 | 123 The number of brands that sold at least one electric vehicle in China last year, according to Stephen Dyer, a Shanghai-based auto consultant at AlixPartners. China has more than 100 domestic brands that churn out more vehicles than the country’s drivers buy each year. Yet the government encourages unprofitable carmakers to keep producing as officials try to boost economic growth, preserve jobs and expand China’s role in the global EV industry. This overcapacity adds cars to a global market that risks becoming more oversupplied. (from WSJ) | ![]() hosede | |
28/4/2024 17:28 | Saw recent controversial football incidents on offside rulings and ball over the line events. So thick is the computer, 'in line' at offside meant just that, the bulk of the mass of one player was in line with the bulk of the mass of the defender. But it cannot handle that, it can only detect that a tiny finger or the slightest part of the attacking player is beyond all of the defender. Same with ball over the line. If the whole mass of the ball is over the line not even touching the line the computer can only handle the projected ball to extreme edge of line. Ridiculous. The fun will start with robot taxis. A straight road from point a to point b ok, maybe, but anything else, no chance. | ![]() careful | |
28/4/2024 17:14 | AI in robo taxis will not only need to achieve reliable image recognition, they will have to predict movements. We get used to this great gift in tennis, football, even when driving. It is very subtle, and hard to believe that AI will ever be able to do it. Anticipation. You know the sort of thing, that dog looks as though it os about to run onto the road...but wait a minute, it is moving towards the road but we can now see that it is tethered to a post. How about nasty kids , pretending to move towards a road for fun, then stopping well short of the kirb. Or a sign, residents only, closed to other traffic. When does a bunch of rowdy thugs look menacing, instinct, and could AI sense it? Love the way the magnificent Alsatian police dogs at the football matches had to be held back from attacking skin head yobs. How did they know and can it be programmed. They were not bothered about well behaved supporters. Beating a grandmaster at chess, well that is simple, just remembering every past move in every situation. It would be very simple to create a long list of things that it will not be able to do. | ![]() careful | |
28/4/2024 15:53 | cfb2 - Personally I think that fusion needs the free work provided by the compression of space time of the mass off a star. Trying to do that with other methods requires vast amounts of energy such that it is difficult to make a surplus, especially given the maximum efficiency of heat cycles. | ![]() hpcg | |
28/4/2024 15:26 | CFb But doesn't more training mean bigger and bigger data banks - as Simon's article above said multiplying the data base by a hundred only improved effectiveness from 65 to 67% or something similar. I presume all this data has to be in each car, as it cannot always be in touch with "Tesla home base" | ![]() hosede | |
28/4/2024 14:20 | Fusion requires new maths to keep the plasma contained (AI has shown to be a promising approach though...) and several breakthroughs so the energy out exceeds energy going in: Qtotal > 1 rather than Qplasma > 1 (Qplasma > 1 has been achieved). Problems are being solved and new approaches are being tried but I'm not aware of any in the public domain that will result in Qtotal > 1. Tesla's FSD requires more training data for their neural networks, which takes time. Tesla is no longer compute constrained but they do need to filter incoming video data sent from cars before using it for training. Remember that Tesla have sight of new releases of FSD many months before it goes to their employees or wider release. For them to be preparing the changes to make robotaxis I'm expecting the driving better than a human to be solved. In other words, the plateauing eluded to by the Will Lockett article occurs at a level sufficiently safe for Tesla to get approval to run the robotaxis service. I'm not expecting the additional stuff to control fleets of robotaxis to be done yet, or by 8/8/24. It's unlikely the FSD software will be approved by then either. | ![]() cfb2 | |
28/4/2024 12:13 | I think FSD like energy from fusion is one of those things that's always "going to happen" but never does - like "free beer tomorrow" | ![]() hosede | |
28/4/2024 11:46 | On the scale at the bottom it says: As of July 2023 So, before Musk demonstrated FSD V12 with end to end neural networks in a live stream (September 2023). I wonder whether Gartner would still think "5 to 10 years" now? Spoiler alert: they would because they produce graphics to demonstrate their paying customers either lead the market or have made a wise decision. Have a read of the following article to explain why Gartner's research is worthless: | ![]() cfb2 | |
27/4/2024 21:01 | LinkedIn - December 2023: Is the Large Language Model revolution just getting started or are we closer to the end? by David Johnston Principal Data Scientist at ThoughtWorks Conclusion I think there is a very good chance, as strange as it sounds, that LLM research has already made the major breakthrough, and that future progress will be incremental rather than revolutionary. AI however is a much bigger field and I expect continued research and progress on all sorts of things. The current paradigm, pattern recognition, will continue to be the leading methodology for perhaps another decade. After that, if there is much progress, I expect it to come from other areas of AI, some of which have fallen out of favor, or just briefly taken a backseat (e.g. reinforcement learning). Or perhaps we will finally figure out how to combine multiple paradigms into a larger system similar to how the human brain appears to work. But LLMs are probably approaching the point of being a solved problem and focus will shift away from making them more “intelligent&r | simon gordon | |
27/4/2024 20:10 | Bluster, Status quo junkies? What's wrong with asking questions? The hype is high from some stock promoters. Has there not been phases in the development of AI when it hit a roadblock and took more time to make the next leap? I think the writer is making this point. | simon gordon | |
27/4/2024 18:59 | Bluster The article suggests that we have reached a theoretical maximum beyond which progress is virtually impossible. | ![]() hosede | |
27/4/2024 18:42 | Simon, This makes the endlessly repeated mistake of saying ‘as things are today, progress can’t be made’. The obvious flaw being ‘as things are today’. Good luck status quo junkies, you’ll be wrong again (and again and again) soon enough.. simon gordon27 Apr '24 - 18:11 - 10697 of 10699 | ![]() blusteradjuster |
It looks like you are not logged in. Click the button below to log in and keep track of your recent history.
Support: +44 (0) 203 8794 460 | support@advfn.com
By accessing the services available at ADVFN you are agreeing to be bound by ADVFN's Terms & Conditions