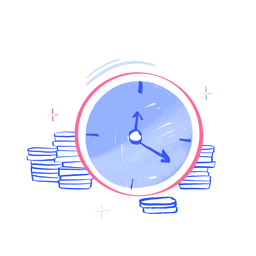
We could not find any results for:
Make sure your spelling is correct or try broadening your search.
Share Name | Share Symbol | Market | Type | Share ISIN | Share Description |
---|---|---|---|---|---|
Physiomics Plc | LSE:PYC | London | Ordinary Share | GB00BDR6W943 | ORD 0.4P |
Price Change | % Change | Share Price | Bid Price | Offer Price | High Price | Low Price | Open Price | Shares Traded | Last Trade | |
---|---|---|---|---|---|---|---|---|---|---|
0.05 | 3.33% | 1.55 | 1.50 | 1.60 | 1.60 | 1.50 | 1.50 | 862,880 | 15:46:32 |
Industry Sector | Turnover | Profit | EPS - Basic | PE Ratio | Market Cap |
---|---|---|---|---|---|
Pharmaceutical Preparations | 597k | -477k | -0.0035 | -4.43 | 2.1M |
Date | Subject | Author | Discuss |
---|---|---|---|
09/7/2020 09:49 | Oh Stig, the king of irrelevant spam. Pyc doesn't use A.I pyc isn't involved in 'drug discovery' Everything you spammed above, pointless. | ![]() davevt | |
08/7/2020 20:21 | Physiomics retweeted this out this week from their partners Merck KGaA -------------------- AI IN DRUG DISCOVERY From Siri to facial recognition, AI and machine learning are becoming familiar features of everyday life. But they could also save lives, by helping find new and better treatments for disease, faster. Back in 2011, IBM’s Watson supercomputer won the US TV game show Jeopardy and captured the public’s imagination. At that time, smartphones had no built-in Siri or Google Assistant and few people would have believed how commonplace devices such as Google Home and Amazon Alexa would become. Watson's win immediately made artificial intelligence (AI) advances tangible for millions of people. There are many different types of AI, but the most common is ‘machine learning’. In machine learning, an input is added – for example, an image. A complex function is then applied to connect that image with an observation – for example, ‘cat’. In the case of the example, you would then have the ability to automatically sift through a collection of images to find images of cats. AI and machine learning are now used in many applications, from the example of image classification above [3] to autonomous driving. But one area of AI research where there are untapped opportunities is looking at how we can apply it to healthcare and drug discovery. Due to an explosion in knowledge about the molecular mechanisms behind different diseases, more promising targets for the design of new drugs are being identified than ever before. But the processes behind drug discovery can be challenging. Can AI and machine learning help alleviate some of the traditional bottlenecks, so we can get new and more effective drugs to patients, faster? DID YOU KNOW? 2.6bn US dollar is the current cost of bringing a new drug to the market.[1] 10% of drug candidates make it to market following Phase I trials.[2] 300 AI predictive models for compound properties have been developed by us.[6] WHY USE AI IN DRUG DISCOVERY? The central goal of drug discovery research is to identify medicines that act beneficially on the body – in other words, they can help prevent or treat a particular disease. Although there are several different types of drugs, many are small chemically synthesized molecules that can specifically bind to a target molecule – usually a protein – involved in a disease. To find these molecules, researchers traditionally carry out large screens of libraries of molecules to identify one with the potential to become a drug. They then go through numerous rounds of tests to develop this into a promising compound. Recently, more rational structure-based drug design approaches are becoming increasingly common. These avoid the initial screening stages but still require chemists to create potential new drugs by designing, synthesizing and evaluating numerous compounds. Because it’s generally unknown which chemical structures will have both the desired biological effects and the properties needed to become an effective drug, the process of refining a promising compound into a drug candidate can be both expensive and time-consuming. Latest figures show that the cost of bringing a new drug to market now averages US$2.6bn.[1] In addition, even once a new drug candidate shows potential in laboratory testing, it may still fail when it is moved into clinical trials. In fact, less than 10% of drug candidates make it to market following Phase I trials.[2] Considering this, it’s not surprising that experts are now looking to the unparalleled dataprocessing potential of AI systems as a way to accelerate and reduce the cost of discovering new drugs. According to market research firm Bekryl, AI has the potential to offer over US$70 billion in savings for the drug discovery process by 2028.[5] HOW CAN AI BE APPLIED TO DRUG DISCOVERY? The sheer size of the libraries used to screen for new drug candidates means it’s now practically impossible for individual researchers to review everything themselves - and that’s where AI and machine learning can help. These sophisticated techniques allow researchers to extract hidden insights from huge datasets. The benefits of doing this are numerous: Predicting the properties of a potential compound, meaning that only compounds with desired properties are chosen for synthesis – saving time and money by preventing work on compounds that are unlikely to be effective. Generating ideas for entirely novel compounds, where the ‘invented&rsqu Alleviating the need for repetitive tasks, such as the analysis of thousands of histology images – saving hundreds of person-hours in the laboratory. These are just a few of the potential advantages, looking at the early end of the drug discovery pipeline. APPLYING DEEP NEURAL NETWORKS IN DRUG DISCOVERY To understand how AI is being applied in practice, let’s take a look at some of the innovative research we currently have underway. “Predictive models are central to our work,” says Friedrich Rippmann, Director, Computational Chemistry & Biology at Merck. “These are statistical models that predict whether a compound idea – a not-yet-synthesized molecule – will produce a desired activity.” “The technologies we’re using mostly relate to machine learning. In particular, we’re using various types of deep neural networks. But we’ve also explored other more classical statistical techniques, with funny names like random forests and support vector machines.” Deep neural networks started to become particularly popular around 2012, when researchers from the University of Toronto won the ImageNet Large Scale Visual Recognition Challenge (ILSVRC).[1] In recent years, this subset of machine learning techniques has revolutionized several fields such as computer vision, natural language processing, and game playing. And now it’s showing exciting promise in identifying novel compounds. “We’ve driving innovative research in the area of interpretable deep neural networks,” continues Rippmann. “Our predictions no longer only tell us, ‘yes, this will work’ or ‘no, it won’t work’. Now they can also tell us why they will or won’t work, by highlighting the areas in a molecule that are responsible for a certain activity. This gives an immediate indication to chemists of what to do to remove a certain unwanted activity.” Their findings are described in greater detail in a recent, well-received article, Interpretable Deep Learning in Drug Discovery.[5] So far, in terms of practical benefit, the research carried out by Friedrich Rippmann’s team and Merck’s partners has made available almost 300 new models for assessing the properties of a compound, which can help predict their ability to bind to a specific disease-relevant target. “These models are already being used by our chemists to judge their compound ideas before deciding on whether to synthesize them,” adds Rippmann. COLLABORATION TO ENHANCE AI IN DRUG DISCOVERY Making advances in this field is, by nature, a collaborative process. “We rarely develop novel methods entirely by ourselves,” says Rippmann. “All our work is done in collaboration with leading academic groups and start-up companies.” So far, notable collaborations include working with Professor Sepp Hochreiter at Universität Linz in Austria – who leads one of the top academic groups in this research area. In addition, we’re also collaborating with Cyclica in Toronto, using their predictive technology that identifies which human proteins a chemical compound is likely to target (for example, in the deconvolution of phenotypic screens). And separately, we’re working with Iktos, a start-up based in Paris, using their technology to automatically ‘invent’ novel molecules that hit predefined requirements for a drug, including binding to the drug target, lack of specific toxicities, solubility, and much more. But collaboration to enhance AI in drug discovery is also happening at a larger scale. AI analysis is only as good as the quality of the datasets in use. For this reason, the pharmaceutical industry is increasingly seeking to collaborate by pooling data. A recent initiative to facilitate, the MELLODDY Project, involves the EU Innovative Medicines Initiative and no fewer than ten pharmaceutical companies – including Merck. It aims at improving predictive models through so-called ‘federated learning’, by using a novel blockchain system to store data on a secure ledger while protecting the trade secrets of individual companies. WHAT DOES THE FUTURE HOLD? The biggest opportunity for the future, according to Friedrich Rippmann, is the acceleration of drug discovery and reduction of attrition rates, ultimately making more novel drugs available to patients, faster. “There are so many opportunities to apply AI in drug discovery,” he says. “But at the moment we’re hindered at times by the prohibitive costs involved. As more competition emerges though, we will see costs coming down – opening up exciting possibilities for new discoveries in diverse fields.” REFERENCES [1] [2] a%3Dihub [3] [4] [5] [6] | ![]() the stigologist | |
08/7/2020 20:12 | After 10 years of building their Virtual Tumour Cancer model Physiomics finally seem set for the big time So far Customers have just been bleeding edge serious innovators like Merck KGaA and Bicycle Therapeutics but eventually ALL BIOS and PHARMAS will have to move to Modelling & Simulation / BioSimulation / InSilico Clinical Trials All the 70+ projects Physiomics have done over the last 10 years with likes of Merck KGaA, MSD, Lilly, Bicycle, CellCentric will give them unique insight into modelling how these drugs work and all incorporated into the Virtual Tumour model The chart shows a long 10 year basing pattern. The November 2017 3000% move just looks like a blip ! I fully expect over the next 10 years Physiomics to become a £100m+ Company (indeed the valuations of their Comparators Simulations Plus $1000m and Schrodinger $6000m demonstrate the potential in selling into the $180 Billion a year Pharma R&D budget) There are about 50 Large Pharmas There are hundreds of Bios and Small Pharmas in Oncology space alone | ![]() the stigologist | |
08/7/2020 20:05 | Setting up beautifully for the next moonshoot Golden Cross + Pennant / Bull Flag + Volume | ![]() the stigologist | |
08/7/2020 19:07 | I take it you're new here lol. Pyc charge 35k per cancer drug, pre clinical. 165 per clinical. obviously clinical doesn't happen until pre clinical stage has passed. Remember, the jobs aren't paying wages, investors are. Hence why the company has never made a profit since inception, and it doesn't have to. Go and look at all the previous rns for a pre clinical job, it's always 35k, and multiples of depending on how many drugs are being dose predicted. | ![]() davevt | |
08/7/2020 17:49 | Davevt - 2 full time clinicians for 6 months is not going to be just £35k. And they wouldn't even mention it in RNSs if it wasn't material | ![]() owenga | |
08/7/2020 17:07 | The Merck deal was an extension of them working for 6 previous years with pyc, and going from pre clinical to clinical stage. They went from 35k pre clinical jobs, to 165k clinical jobs. On only 3 cancer drugs, in 6 years, hence why it was 500k euros. Any new customer is starting right back at the beginning, at 35k contracts, if pyc are charging them at all. | ![]() davevt | |
08/7/2020 13:31 | Two nice delayed buys printed as sells. Nice work MMs | ![]() spudyoulike | |
08/7/2020 13:02 | Let's hope so. Been in here quite some time with an average of 10p. I think 20p is very doable on the next news. | rolaw1 | |
08/7/2020 13:01 | Gaining some traction Stig!! | ![]() ant15 | |
08/7/2020 13:00 | Leaked news 20p here | ![]() spacedust | |
08/7/2020 12:58 | Added a few more here. Has potential if it can get back above last weeks high. | matt123d | |
08/7/2020 12:46 | When you strike deals with Big Pharma you have to adhere to their timelines As PYC get more used to agreeing such deals they and their lawyers get more used to finessing and expediting deals. The Merck KGaA deal was flagged Oct 2nd and delivered Nov 28th The current Large Pharma deal was flagged May 27th and we're now July 8th. Suspect we should have news within weeks However we are not just dependent on one news item. Hybridan the Company Brokers have said there are "multiple potential catalysts for share price appreciation" Our peers Simulations Plus and Schrodinger are valued at 30x and 70x revenue which could value us at 30-70p ! | ![]() the stigologist | |
08/7/2020 12:46 | Yep it's imminent territory so anytime time the RNS should land.. | ![]() zen12 | |
08/7/2020 12:43 | Zen12 it is looking good and is trading at levels constantly higher than the placing Perhaps we will be favoured with an announcement shortly that will define where this company deserves to be. So much promise!! | ![]() ant15 | |
08/7/2020 12:24 | RNS please...must be extra imminent being a week now since RNS confirming talks in final stages. | ![]() zen12 | |
08/7/2020 12:15 | SPQStrader get in and buy some more then I have just topped up again. | ![]() ant15 | |
08/7/2020 10:52 | With all the incoming contracts and confirmed pending substantial one with large pharma client, we should be generating between 1.5 - 2 million in revenue this year. With a market cap of only just over 5 million at the moment, that is WAY UNDERVALUED compared to our peers. If we are valued at a minimum of 10x revenue, that equates to a value of minimum 20p from here. And that's without any other new contracts potentially in the pipeline that we are unaware of at present. PYC is much less of a risk now that we have an II on board. There is a reason they took part in the recent fundraise.... | spqstrader | |
07/7/2020 19:43 | Stig, pyc don't do 'drug development'. By the time pyc would be involved, the drug is already developed. | ![]() davevt | |
07/7/2020 19:00 | Yet the price keeps going down . Imagine world class pharma didn't mention PYC the what the price would have gone down to | ![]() spacedust | |
07/7/2020 14:02 | Jut Physiomics' work being cited by one of the biggest Global Pharmas US Merck/MSD (MRK) "QSP tools are the foundation of the model‐informed drug development paradigm at Merck for integrating knowledge, enabling decisions, and enhancing submissions. Rigorous prioritization of M&S activities has enabled key drug development decisions and led to high ROI" | ![]() the stigologist | |
07/7/2020 13:05 | These people look about 12 :-D | ![]() the stigologist | |
07/7/2020 10:33 | Very interesting tweet out from PYC Oxford University AI initiative with Roche and GE Dr Timor Kadir, Chief Science & Technology Officer at Optellum Ltd, commented, 'Three industry leaders - Roche, Optellum and GE - have joined their expertise in molecular diagnostics, imaging and AI to help diagnose and treat lung cancer patients at the earliest possible stage. The programme results will be integrated into Optellum’s AI-driven Clinical Decision Support platform that supports physicians in choosing the optimal diagnostic and treatment procedures for the right patient at the right time.' Ben Newton, General Manager, Oncology, at GE Healthcare, said, 'We are very pleased to be working with the University of Oxford via the NCIMI project on this important lung cancer research. By extending our existing NCIMI data infrastructure and creating innovative AI solutions to spot comorbid pathologies, we aim to help identify lung diseases earlier in the UK.' Geoff Twist, Managing Director UK and Ireland and Management Centre European Agents at Roche Diagnostics Ltd, said, 'We are thrilled with this funding award, because it gives us the opportunity to work towards ground-breaking innovation in early diagnosis and because working in partnership is vital to achieve success in the health system. By bringing together the collective knowledge and expertise of these academic, medical and industry partners, this project has the potential to impact patient care globally through new diagnostic solutions in lung cancer.' | ![]() the stigologist | |
06/7/2020 22:49 | 1. who will the big pharma be? It doesn't matter. We've had big pharmas before and it didn't mean Jack Schmidt. They could even announce a undisclosed pharma 2. How much will the contract be? I asked many times what figures people are expecting. I'm expecting undisclosed amount which means zero 3. Any hints to repeat business. Again doesn't matter they'd be undisclosed fee repeat business. We've had repeat business before | ![]() spacedust |
It looks like you are not logged in. Click the button below to log in and keep track of your recent history.
Support: +44 (0) 203 8794 460 | support@advfn.com
By accessing the services available at ADVFN you are agreeing to be bound by ADVFN's Terms & Conditions